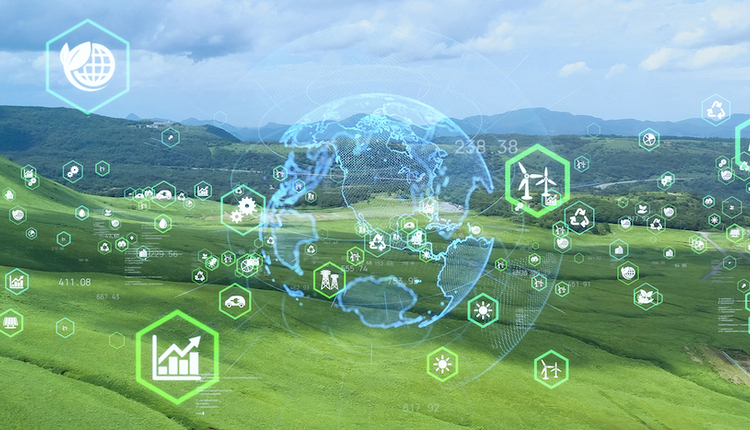
In 2020 electricity production created 25% of global greenhouse gases directly and indirectly through powering industry another 21% (epa.gov). Fossil fuels created 63% of that (ourworldindata.org). In response, electric utilities (EUs) are migrating from fossil sources like natural gas, oil and coal to green ones like wind, water and solar. But it’s a slow go, and it’s not for lack of pollution regulations — globally, they’ve ballooned many-fold since the ‘70s. Recently, most countries at COP26 in 2021 pledged to meet The United Nations’ Sustainable Development Goals for 2030. Pledges made at the 2016 Paris Agreement, however, were largely unmet. That does not bode well for 2030.
Regulations and pledges are one thing — reality is another. Environmental law is poorly enforced — this is the key reason that global pollution increases unabated.
Citizens, though, are demanding change. With data-driven tech, EUs may digitally transform and disrupt energy as we know it.
EU Types and Markets
Electric utilities generate, transmit and distribute electricity for sale in regulated and deregulated markets. There are four types: investor-owned utilities (IOUs) issue stock to shareholders; public ones are run by federal, state and municipal governments; cooperatives are rural, not-for-profit and member-owned; nationalized are owned by countries and run as virtual monopolies.
In US regulated markets, utilities own all infrastructure and sell at rates set by state commissions to consumers locked into that market. In deregulated markets, participants invest in the infrastructure, and utilities generate and sell electricity wholesale to suppliers that buy and sell it at competing rates. Regulated markets offer stable, predictable rates over a long term; deregulated ones offer competitive rates, so customers have more choice, but rates fluctuate. Regulated markets are by nature slow to change, so they lag deregulated ones in adoption of green tech, but both are tending greener. In both, reliability is paramount. A power outage at a big EU can, for instance, crash manufacturers’ production lines and hospitals’ vital operations – while it kills the lights for hundreds of thousands of customers.
Slouching Towards Green
Because EUs now get data from both fossil and greentech sources upstream and from residential, commercial and industrial customers downstream, they are drowning in a dynamic soup of omnidirectional megadata from Internet-of-Things (IoT) sensors at power sources and in cities and factories as well as from solar panels on customers’ houses and buildings. That quantitative increase of data is forcing qualitative change. EUs are becoming digital data ecosystems in which contenttech is indispensable and which more comprehensive IT force-multipliers will superempower.
- Artificial intelligence (AI) will holistically enhance content and other tech throughout EUs.
- Software-as-a-Service (SaaS), particularly Business Process-as-a-Service (BPaaS), will cut capital expenses, speed deployment, eliminate troubleshooting and management and let users collaborate over processes in discrete apps like human resources remotely from anywhere.
- Superchip-enabled clouds will be pay-per-use and megapower-on-demand for dynamite, but affordable, performance.
- Data lakes and data mesh will decentralize data ownership so workers with use case expertise can better service customers.
- No-code/low-code will simplify app development so it’s more ubiquitous.
- Superchips with trillions of transistors per wafer will power analysis of huge AI data sets to yield much better business intelligence (BI) that EUs can leverage for tech innovation and customer engagement.
Before that can happen, though, EUs must undergo structural change.
An Aspirational Architecture
The emerging standards-compliant, open architecture will support record, intelligence and engagement systems. The first will be comprised of legacy apps like ERP and those controlling interoperability with the electricity grid; the second of customized ones like contenttech and e-commerce that modernize and differentiate the EU by tech innovation; the third of apps like marketing technology and customer experience that promote EU services and provide insights into customer behavior for enhanced customer service. They’ll do app development faster as it will be component-based; they’ll minimize resource management as servers will reside in the cloud but available on demand. It’s likely vertical integration of the three systems and apps will require a systems integrator (SI), though integration hubs exist to expedite it, and it will happen in a domain-specific integration-Platform-as-a-service (iPaaS). The iPaaS allows no-/low-code development, execution and governance of integration flows linking on-prem and cloud apps and services.
The Power of AI
Databloat is a curse and blessing for EUs because the data soup they are drowning in will also provide the big data sets AI will train on. Generally, the bigger the data set, the better the business intelligence that AI can extract from it. It’s not feasible to analyze all of EUs’ ever-growing data soup, but EUs can analyze bigger data sets within it. Earlier iterations of BI inform the AI used later to analyze a set, so resulting data models are constantly retrained. Ever-better models yield ever-better insights, and those insights loop back and refine ever-growing data sets.
AI data pipelines ingest real-time and historical data streaming from the interprise edge and omniforms of voice, video, email, text files, etc. throughout interprise. Data scientists label the datasoup by cleansing the noise (purposeless data like duplicates, etc.) and reconciling file formats (JPEG, MPEG, etc.). Dashboards surface and visually present for end users the characteristics (prospects per sales channel, etc.) of the resulting betterdata. This process yields an aggregate of constantly refined betterdata, or data lake.
Traditional AI is model-driven: data scientists create then train and retrain data models to better parse the data comprising the data lake for better BI. Newer AI is data-driven: scientists spend less time creating good enough (not constantly retrained) data models and instead hyperprep the data in the data lake so it informs the model. In the first case, humans do most of the data labeling; in the second, AI does it. Both improve the quality of data, but data-driven AI improves the data and model simultaneously, and that bidirectional synergy self-actualizes the data for much faster time-to-quality.
Data lakes are tech-centric, monolithic and centralized because data scientists enrich data primarily as they are influenced by C-level managers with nominal input from domain experts, so data is nominally differentiated in the data lake and ownership resides in executives high up in the interprise hierarchy. Data meshes are organizational principles that reverse this dynamic. They reorganize data by business units, which makes data lakes business-centric, data highly differentiated and ownership decentralized and down in the hierarchy. Data is thereby focused on specific use cases, so it can inform better customer service and be marketed and sold as a product.
AI will lower costs, increase revenues from data as a product, improve productivity and speed payback as well as expedite decision-making, enable tech and customer service innovation, allow performance monitoring and quicken response to unforeseen business problems. These benefits will make EUs more adaptable to the flux of new tech and market forces.
The Role of Contenttech
Knowledge workers spend the equivalent of a day a week searching for information. EUs are especially data access- and management-challenged for many reasons.
- They deal with ever-changing big data.
- That data is omniform.EU terminology pervades it.
- It’s siloed in legacy and outsourced apps which hampers findability.
- It’s taxonomized by manual or sloppy digital methods.
- Therefore, north of 90% is dark so inaccessible to, say, line-of-business users who could otherwise leverage it for better customer service.
Contenttech Can Solve These Problems
Intelligent enterprise search locates and indexes documents in different formats like PDF and JPEG. With Natural Language Processing (NLP), it continuously learns based on previous queries, so it nets increasingly focused results even as data constantly streams through the EU. These inform customer profiles so reps can personalize service and be more productive in the process.
With data analytics, EUs can perform proactive customer service. After storms, analytics detect interrupted IoT data streams from field equipment that indicate failures. Service teams then quickly address them, so customers experience less downtime. Analytics also let EUs operate more efficiently. Analytics cluster customers who have similar usage patterns — say, heavy use when kids come home from school — so EUs can provision to the right group at the right time and pass on the savings to customers. Analytics via smart meters automatically and wirelessly provide real-time usage and cost data to in-house displays so customers’ can monitor and economically manage consumption. EUs render data thusly as value-added services they can charge for.
Typical EUs have of hundreds of ad hoc, redundant and inefficient manual and automated business processes in a spaghetti-like mashup resulting from upgrades and replacement of legacy apps like enterprise resource planning (ERP), customer relationship management (CRM) and supervisory control and data acquisition (SCADA) — for machine control — and asset management — for, say, field equipment, as well as a patchwork of modernizing apps like Internet-of-Things (IoT), auto-meter-reading, customer service portals, each with its own processes islanded from legacy ones. Workers also improvise manual workarounds that create shadow processes. The result is a process mess, and managing it requires the majority of IT’s budget better dedicated to core business.
Interview-based discovery of, and manual efforts to streamline these, are incredibly time-consuming and expensive.
Cloud-based business process management (BPM) lets EUs discover, model, automate, monitor and continually improve their processes. They can find on-premises and cloud “as is” shadow, manual and automated processes, optimize processes with high business value, retire low-value or redundant ones, and consolidate others so they’re more efficient. Because infrastructure and operations are in the cloud, the solution requires little up-front capital, the provider sets up, maintains and troubleshoots it much easier and faster than if it were installed, its pay-for-use cost model makes it very affordable, and it scales as needed as the EU modernizes. Users then get anytime/anywhere remote collaboration over frictionless processes linking legacy and differentiating apps and even those tracing the customer’s journey. Cloud-native records, document and content management come provided to various degree and power by enterprise content management (ECM) platforms. ECM is strong on records auditing, compliance and cradle-to-grave content management. Some vendors can deploy in multi-cloud environments for scale; some even specialize in regulated industries (especially relevant to EUs).
New Models for a New Age
EUs will adopt new business and tech models to better interact with increasingly greentech-empowered energy sources and customers. As contenttech has become more virtual and powerful when complemented by AI and on the cloud, it will promote EUs’ digital transformation now required as ante to play in the new hybrid energy era.John Harney is President of SaaSWatch, where he consults on Software-as-a-Service techs and markets. He also reports on IT issues across most industries, particularly where SaaS, cloud, AI and content are solutions, and especially as they drive the greening of the planet. He can be reached at 240.877.5019 and jharney583@gmail.com.