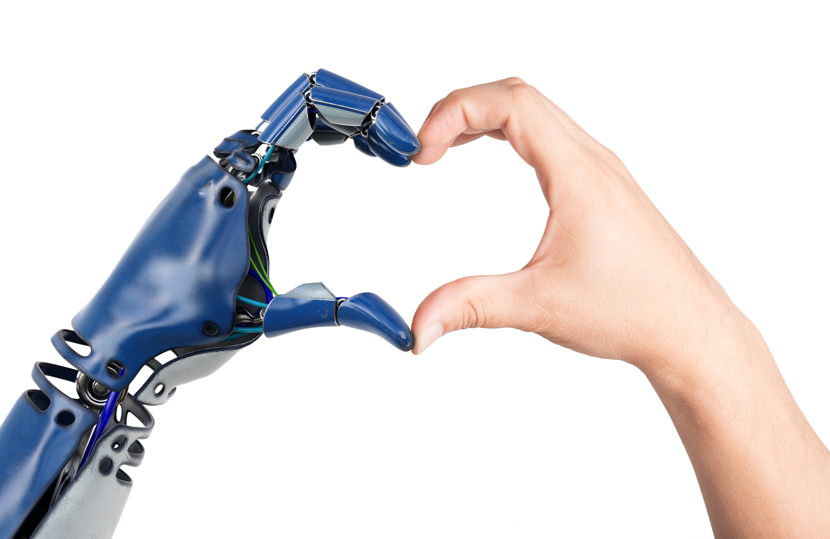
Image by: dimdimich, ©2018 Getty Images
Robotic process automation (RPA) is the latest solution category promising an easier path to digital transformation. In the simplest of terms, if you have a process that is well-defined, RPA can automate it. Over the past year, an interesting variation of RPA has begun to surface called cognitive RPA. So, what exactly is cognitive RPA, and how is it different from standard RPA technology?
First, it's important to understand what RPA actually does. RPA automates tasks that are highly standardized and involve structured information. It does so with what are typically called "bots," which use recordings of particular staff members executing a given activity—for instance, the provisioning of an email account for a new employee.
Using the above example, this is typically done through a graphical user interface (GUI). A member of the information technology (IT) department will enter the name of the employee and set various parameters, such as mailbox size, memberships to groups, etc. Using RPA, a person will initiate a session that records the activities of IT staff, what data to enter, what options to select, and then stores it in a library of tasks.
The key to successful RPA projects is to select tasks that have little variation and few chances for exceptions. However, those tasks involving unstructured information, such as data stored within documents, have been out of reach for this technology set until the arrival of cognitive RPA.
Unstructured Data & Cognitive RPA
In most circles, this ability to use more complex data is called cognitive RPA. The term “cognitive” means that these systems should be able to deal with unstructured data in the same manner in which a human would (such as deploying chatbots for basic customer assistance tasks that would normally require a human staff member).However, the reality is that unstructured data is complex and the ability to use this information—whether through data extraction or gleaning insights from it—is much more difficult than using structured information. Most RPA vendors still think of basic zoned optical character recognition (OCR) as part of a cognitive RPA solution.
There is also a challenge with managing expectations. Unlike traditional RPA—which can have close to 100% automation—cognitive RPA processes involving unstructured information have a much higher percentage of exceptions that require manual review.
For example, let’s look at that email account provisioning task we mentioned earlier. Within an organization, it's possible to have a well-defined set of tasks using only structured information in order to automate provisioning a new email account. Starting with a form that asks a new employee to provide name, phone number, and department, an RPA bot can use the email system to create a user with little chance for a problem arising.
RPA: Unattended Mode
To better define the differences, consulting firm Everest Group draws a distinction between traditional RPA solutions, which operate in “unattended mode,” from complex, unstructured data processes that require humans in the loop.For example, let’s look at that email account provisioning task we mentioned earlier. Within an organization, it's possible to have a well-defined set of tasks using only structured information in order to automate provisioning a new email account. Starting with a form that asks a new employee to provide name, phone number, and department, an RPA bot can use the email system to create a user with little chance for a problem arising.
Now, take that same task but allow a new employee to provide this information over a phone call or through an interactive chat session, and the ability to collect the correct information (let alone use it) can become very complex.
Going even further, let's look at a loan approval process where a loan officer must verify all paperwork is submitted and review many different and complex documents. Information within each document is then used to determine the credit worthiness of a customer, typically using loan origination software.
An objective of RPA in the loan scenario is to accelerate the process by automating much of the manual tasks associated with getting the required information into an origination system, including sorting and identifying documents, paging through them, and noting or recording specific data. The sheer number of potential variants in document type and the order in which they are received, coupled with different business rules or conditions (depending on document type), makes using traditional unattended RPA very difficult, if not impossible.
How to Best Apply Document Automation
To really provide automation in complex workflows involving document-based information, we need to re-think how to apply document automation in a manner that allows the overall process to adapt and refine. As document automaton software evolves, more functions will be overtaken by machine learning algorithms that can configure themselves and learn from their mistakes.For example, a self-learning system only needs access to tagged information in order to automate complex and time-consuming tasks, such as analyzing documents and creating rules to identify them or locating needed data. This same system can also use run-time operations to collect background data in which to update configurations, all without the need for manual input. This self-learning ability is not only beneficial, it is mandatory in a work process that is highly varied and could potentially change from one day to another.
Unfortunately, such advanced capability is a far cry from today’s current state of document automation that relies upon zoned coordinates or the creation of specific rules—especially those that are built into RPA solutions—so expect challenges with achieving high rates of automation.
Ultimately, as cognitive RPA matures, high levels of automation with unstructured data can be achieved, but managing expectations of unattended automation is important. More sophisticated processes involving complex, document-based information need to target a mode where humans and bots work together—with bots taking most of the easy work and leaving humans to ensure that everything conforms to expected results.
Due to the complexity of unstructured data, perfection is not realistic, leaving the need for these processes to still be attended at some level by humans.
Greg Council is Vice President of Marketing and Product Management at Parascript. Greg has over 20 years of experience in solution development and marketing within the information management market, including search, content management, and data capture for both on-premise solutions and SaaS.